Do you know what old-as-the-world farming and cutting-edge machine learning share in common? If you thought that all the milking machines, tractors, and harvesters were all the contemporary world of technology was capable of in farming, these amazing uses of machine learning and artificial intelligence in agriculture will totally blow your mind. Now that “smart farming” and “ag-tech” have become the new buzzwords, it’s thrilling to know how the artificial brain is challenging the prehistoric human skill.
Artificial Intelligence and Machine Learning in the Industry of Agriculture
Whatever you call it — digital agriculture, smart farming or agritech — it is all about the use of innovative approaches of data science, artificial intelligence (AI), and machine learning (ML) that help make agriculture processes more effective and produce more using less.
The use of AI in agritech is broad. It is mostly focused on applying machine learning algorithms to help agricultural operational systems learn from new data and improve the experience based on it. Machine learning and AI in agtech are aimed to optimize production by minimizing the risk of human error and gradually reducing the need for human assistance.
Smart Applications of AI and ML in Agriculture
Agriculture ML projects allow sensors and robots to not only collect quality data but also measure it and use this knowledge to, for example, predict yield, detect diseases or weeds, keep track of water levels and soil quality, etc.
At the moment, the use of AI and ML in agriculture is spread unevenly. Sectors that benefit the most from innovative AI/ML technologies are:
- Managing crops
- Managing soil and water condition
- Managing livestock
Let us have a look at how machine learning is used in these niches.
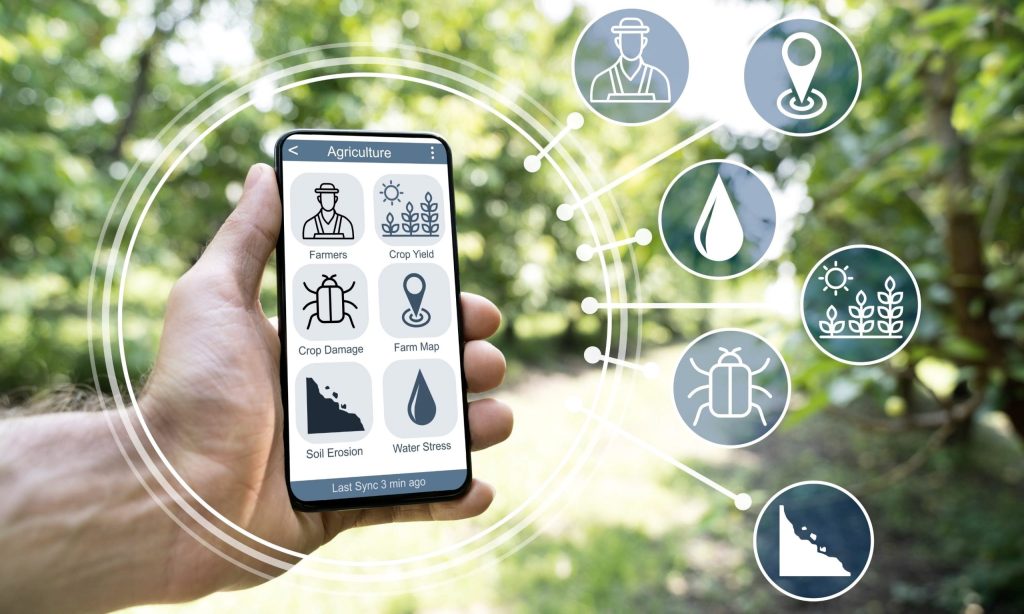
Machine Learning in Agriculture: plant management
Machine learning is already a big part of processes like preparing the soil, breeding seeds, harvesting plants like rice, using computer vision, and determining produce ripeness following special algorithms.
- Species breeding. Algorithms analyze how a certain crop performs in different climate conditions and what new characteristics it develops in the process to predict what kind of genes would be the most beneficial for it.
- Species recognition. Unlike the old-school classification performed by people where they compare colours and shapes of leaves, ML analyzes the morphology of the leaf vein, and therefore, brings quicker and more exact results.
- Crop quality. Artificial intelligence agritech solutions help identify the quality of a crop depending on the chemical analysis of samples. It helps farmers minimize waste and boost the product price.
- Yield prediction. Instead of turning to historical data, machine learning uses computer vision to deliver real-time analysis that takes weather and the condition of the soil into account to provide the most accurate yield prediction.
- Detecting diseases and unwanted weeds. ML relies on image processing that helps accurately recognize weed species that infest the crops, along with a crop disease or deficiency of nutrients, which increases the efficacy of controlling diseases while making them less costly.
- Berry production. By accurately estimating and predicting weather conditions, ML algorithms provide valuable foresight into how the production of different kinds of berries can be optimized. Accurate predictions enable agronomists to modify the process of growing to achieve better results.
Machine Learning in agriculture: resource management
- Managing soil. ML and artificial intelligence in agriculture develop algorithms that help analyze and predict soil properties such as moisture, temperature, drying, and composition. This low-cost and fast approach gives farmers actual knowledge about climate change and helps make more accurate crop yield forecasts for a particular region.
- Water management. AI/ML-based algorithms help use systems of irrigation more effectively and save water by predicting the dew point temperature and evapotranspiration (soil and plant transpiration) on a regular basis.
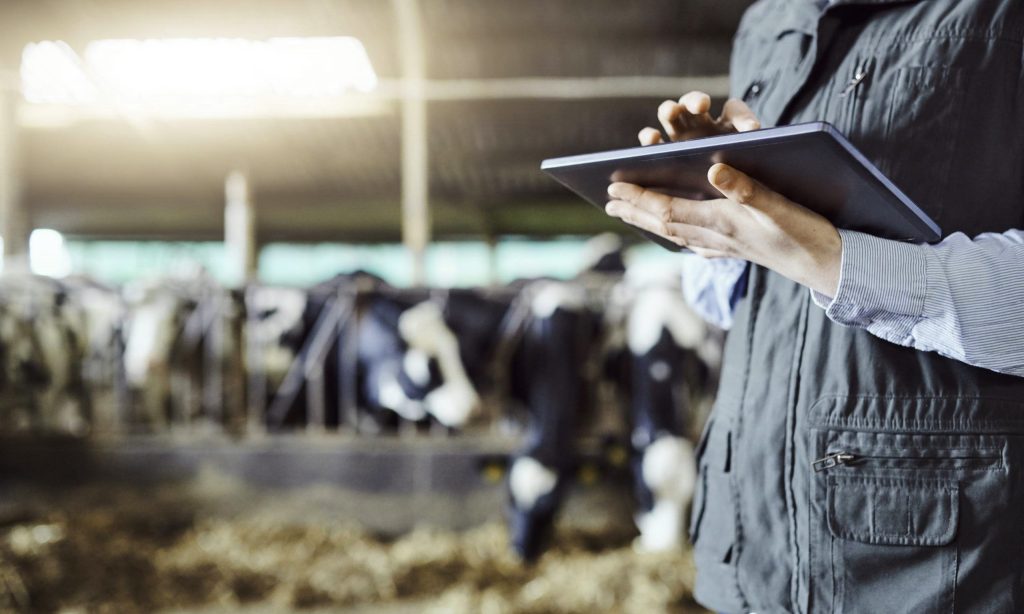
Machine Learning in Agriculture: livestock management
ML-driven algorithms help farmers and ranchers achieve better results in animal herding, selective breeding, milking, dairy production, and, of course, animal welfare.
- Animal Health. Agricultural AI/ML-based systems are applied in monitoring animal behaviour, learning from normal patterns to detect ailments and even predict how susceptible they are to different illnesses. For example, animal behaviour algorithms analyze data collected from video cameras and collar sensors to link a certain type of behaviour with stress or a need for a diet change.
Machine learning and agritech are fantastic examples of how computer science can help solve global social and economic problems. Increased demand for food and a growing population at one end of the scale and climate change at another are what agritech is to balance in the nearest future.
ABNK has implemented AI solutions in our current project, so we can totally recommend including AI or ML in your Agriculture software.